Home
Accelerated Optimization for Machine Learning: First-Order Algorithms
Loading Inventory...
Barnes and Noble
Accelerated Optimization for Machine Learning: First-Order Algorithms
Current price: $169.99
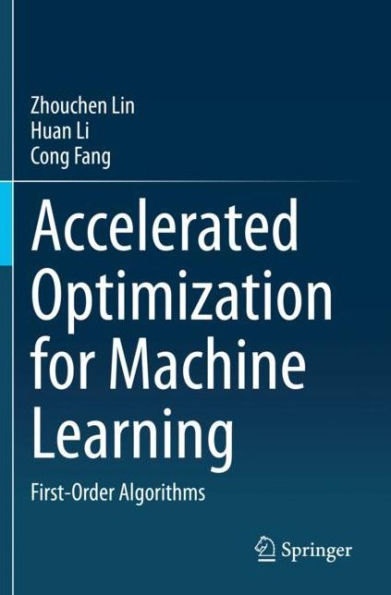
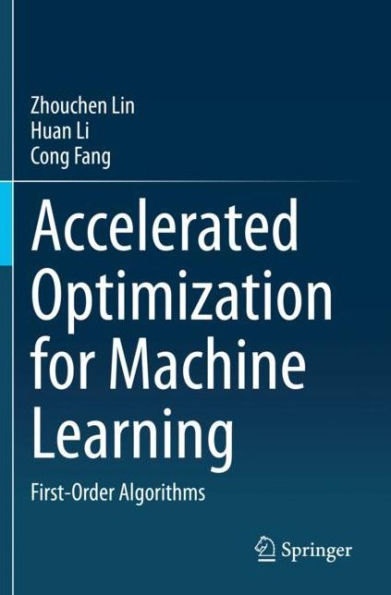
Barnes and Noble
Accelerated Optimization for Machine Learning: First-Order Algorithms
Current price: $169.99
Loading Inventory...
Size: OS
*Product Information may vary - to confirm product availability, pricing, and additional information please contact Barnes and Noble
This book on optimization includes forewords by Michael I. Jordan, Zongben Xu and Zhi-Quan Luo. Machine learning relies heavily on optimization to solve problems with its learning models, and first-order optimization algorithms are the mainstream approaches. The acceleration of first-order optimization algorithms is crucial for the efficiency of machine learning.
Written by leading experts in the field, this book provides a comprehensive introduction to, and state-of-the-art review of accelerated first-order optimization algorithms for machine learning. It discusses a variety of methods, including deterministic and shastic algorithms, where the algorithms can be synchronous or asynchronous, for unconstrained and constrained problems, which can be convex or non-convex. Offering a rich blend of ideas, theories and proofs, the book is up-to-date and self-contained. It is an excellent reference resource for users who are seeking faster optimization algorithms, as well asfor graduate students and researchers wanting to grasp the frontiers of optimization in machine learning in a short time.
Written by leading experts in the field, this book provides a comprehensive introduction to, and state-of-the-art review of accelerated first-order optimization algorithms for machine learning. It discusses a variety of methods, including deterministic and shastic algorithms, where the algorithms can be synchronous or asynchronous, for unconstrained and constrained problems, which can be convex or non-convex. Offering a rich blend of ideas, theories and proofs, the book is up-to-date and self-contained. It is an excellent reference resource for users who are seeking faster optimization algorithms, as well asfor graduate students and researchers wanting to grasp the frontiers of optimization in machine learning in a short time.