Home
Computation of invariant measures with dimension reduction methods
Loading Inventory...
Barnes and Noble
Computation of invariant measures with dimension reduction methods
Current price: $56.00
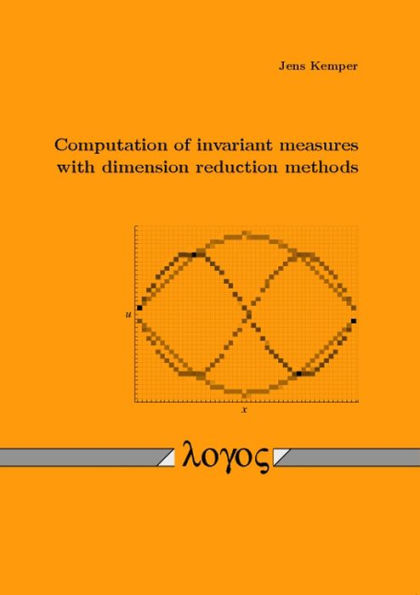
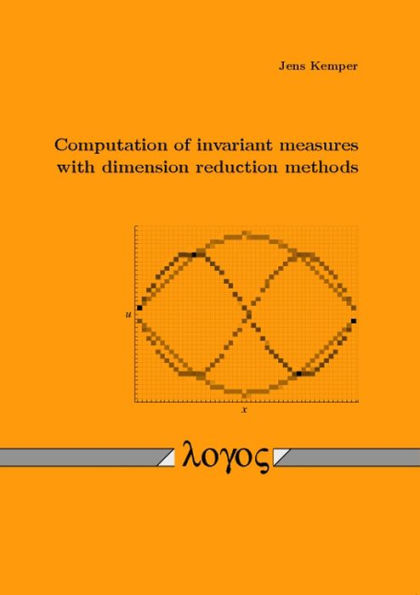
Barnes and Noble
Computation of invariant measures with dimension reduction methods
Current price: $56.00
Loading Inventory...
Size: OS
*Product Information may vary - to confirm product availability, pricing, and additional information please contact Barnes and Noble
In recent years, Dellnitz, Junge and co-workers developed a subdivision algorithm for the approximation of invariant measures in discrete dynamical systems based on the so-called Ulam's approach. In high dimensions, this adaptive invariant measure (AIM) algorithm suffers from the "curse of dimension" even when the support of the system's invariant measure is known to be low-dimensional. In our thesis we develop algorithms facing this problem by combining the subdivision technique with proper orthogonal decomposition (POD) as a model reduction method. We derive explicit error bounds concerning the long-time behavior of POD solutions, propose a discrete version of the Prohorov metric as a proper distance notion for discrete measures computed by the algorithms, and analytically compare the approximation processes of the AIM algorithm and the POD-based algorithms. A marginal-like representation of discrete measures is proposed in order to visualize the numerical experiments. The algorithms are applied to finite element discretizations of the Chafee-Infante problem in order to show the power of our approach.