Home
Perspectives of Neural-Symbolic Integration / Edition 1
Loading Inventory...
Barnes and Noble
Perspectives of Neural-Symbolic Integration / Edition 1
Current price: $219.99
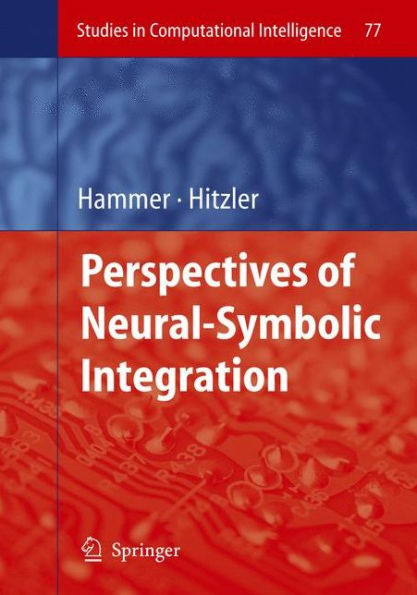
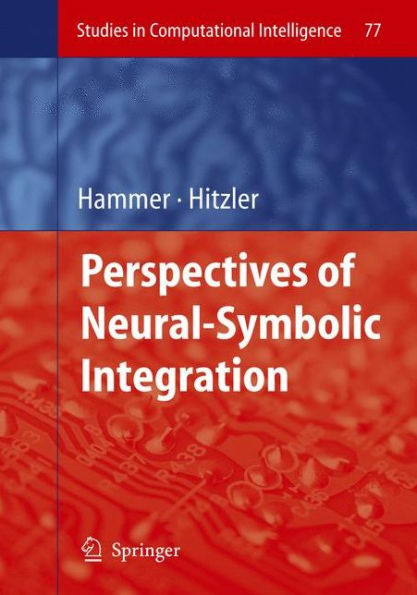
Barnes and Noble
Perspectives of Neural-Symbolic Integration / Edition 1
Current price: $219.99
Loading Inventory...
Size: OS
*Product Information may vary - to confirm product availability, pricing, and additional information please contact Barnes and Noble
The human brain possesses the remarkable capability of understanding, - terpreting, and producing human language, thereby relying mostly on the left hemisphere. The ability to acquire language is innate as can be seen from d- orders such as specific language impairment (SLI), which manifests itself in a missing sense for grammaticality. Language exhibits strong compositionality and structure. Hence biological neural networks are naturally connected to processing and generation of high-level symbolic structures. Unlike their biological counterparts, artificial neural networks and logic do not form such a close liason. Symbolic inference mechanisms and statistical machine learning constitute two major and very different paradigms in ar-cial intelligence which both have their strengths and weaknesses: Statistical methods offerexible and highly effective tools which are ideally suited for possibly corrupted or noisy data, high uncertainty and missing information as occur in everyday life such as sensor streams in robotics, measurements in medicine such as EEG and EKG, financial and market indices, etc. The m- els, however, are often reduced to black box mechanisms which complicate the integration of prior high level knowledge or human inspection, and they lack theabilityopewitharichstructureofobjects,classes,andrelations. S- bolic mechanisms, on the other hand, are perfectly applicative for intuitive human-machine interaction, the integration of complex prior knowledge, and well founded recursive inference. Their capability of dealing with uncertainty andnoiseandtheirefficiencywhenaddressingcorruptedlargescalereal-world data sets, however, is limited. Thus, the inherent strengths and weaknesses of these two methods ideally complement each other.